Applications of systems approaches in the study of rheumatic diseases
Article information
Abstract
The complex interaction of molecules within a biological system constitutes a functional module. These modules are then acted upon by both internal and external factors, such as genetic and environmental stresses, which under certain conditions can manifest as complex disease phenotypes. Recent advances in high-throughput biological analyses, in combination with improved computational methods for data enrichment, functional annotation, and network visualization, have enabled a much deeper understanding of the mechanisms underlying important biological processes by identifying functional modules that are temporally and spatially perturbed in the context of disease development. Systems biology approaches such as these have produced compelling observations that would be impossible to replicate using classical methodologies, with greater insights expected as both the technology and methods improve in the coming years. Here, we examine the use of systems biology and network analysis in the study of a wide range of rheumatic diseases to better understand the underlying molecular and clinical features.
INTRODUCTION
The past few decades have produced substantial insights into the understanding of complex human diseases, due in part to advances in biological techniques. Each new advance provides a different prospective from which to interpret the mechanisms underlying disease phenotypes, leading to the establishment of new criteria for the diagnosis and treatment of specific diseases. On the basis of these scientific achievements, new drugs have been developed and considerable progress in patient care has been made; however, substantial unmet clinical needs remain. As more and more data continue to be produced, this robust accumulation of knowledge often makes it more difficult to prioritize information based upon the importance of that information for laboratory and clinical practices. As new data often show that a disease is more complicated than originally anticipated, a systematic distillation and organization of these data beyond a simple piecing together of a puzzle are required.
Indeed, a prevailing concept in many complex diseases such as diabetes, cancers, autism, and rheumatoid arthritis (RA), is that disease phenotypes arise as a consequence of interactions between genetic, environmental, and lifestyle factors, most of which have not been fully identified [1]. Many complex diseases are classified according to broad, well-established criteria, which are then used to guide treatment. However, this approach often encompasses a heterogeneous disease population exhibiting similar phenotypic traits, regardless of the underlying causes. Two of the best examples of this underlying heterogeneity include cellular heterogeneity within tissues, and genetic heterogeneity across patients [2]. Cellular heterogeneity refers to the differences of aberrant cells and their distinct molecular signature according to the nature of disease, whereas genetic heterogeneity refers to the discordance of dysfunctional genes across patients. Differences such as these are often overlooked using classical reductive approaches which focus on a specific aspect, subsystem, or narrowly defined question, and are therefore ill suited for addressing complex diseases involving nonlinear dynamical interactions among genetic, cellular, and environmental factors.
SYSTEMS-BASED APPROACH TO COMPLEX DISEASES
The rise of the systems approach to human diseases
Classical biology is often defined by its reductionist approach in which a molecule of interest (gene, protein, etc.) is isolated and characterized as a means of determining its role in a particular disease. However, within a living organism these molecules rarely act in isolation, working both cooperatively and antagonistically with other molecules. Furthermore, these interactions can be far reaching, affecting processes not only within the cell, but also in other cells, and even across organs [3]. The degree and range of cellular and molecular disturbances in complex diseases evolves dynamically alongside pathologic changes in related tissues or organs over time. This combination of both clinical factors and biological changes further adds to the complexity and diversity of the disease. However, classical mathematical biology, which focuses on the study of particular functions (e.g., cell growth, differentiation, and proinflammatory activity) of molecular pathways through small-scale modeling and prediction, inherently lacks the analytical power necessary to address such problems.
In recent years, unprecedented advances have been achieved by applying high-throughput technologies and computational approaches to the study of biological systems. Methods such as genome-wide association studies (GWAS), next-generation sequencing, mass spectrometry, and nuclear magnetic resonance have enabled the acquisition of a massive amount of genomic, transcriptomic, proteomic, and metabolomics data, which have been used to identify key determinants of complex diseases [4,5]. As a result, large amounts of data, including sequence alterations and multilevel biomolecular profiles, are available for many human diseases, most of which are accessible from specialized databases and public repositories [6]. The increasing accuracy of these data, along with falling costs, and a reduction in processing time necessary for the acquisition of large-scale omics data have dramatically increased the availability of data for both diseased tissues and specific perturbations [7]. Among the key challenges presented by this newfound wealth of information is the integration of these multiple dimensions to identify associations with distinct clinical phenotypes, and biomarker panels for use in clinical practice.
To handle these large data sets, a systems-level approach is necessary. Systems biology aims to organize and interpret large volumes of highly complex and heterogeneous information generated by high-throughput technologies into a manageable format, to understand how biological systems function [8]. Systems biology first approaches large-scale data through the use of unbiased systematic measurements without a prior hypothesis, and then provides a framework for assembling models of biological systems [2]. Integrated systems analysis networks illustrate the modularity of biological interactions. In this instance, "modules" are defined as collections of genes/proteins or interacting complexes that are involved in the determination of specific cellular functions through network formation [9,10]. They may differ depending on the networks or nodal proteins involved in functional interactions, and transform into a different type through a change in composition, signal integration, and over time. A disease module is based on the "local hypothesis," which states that proteins involved in the same disease have an increased tendency to interact with each other [3]. Disease phenotype can be determined when the critical module is disrupted as a result of any combination of perturbed molecular components.
Systems biology of human disease, also called network medicine, is an emerging tool using both integrative and systems-level approaches for the interpretation of genome-scale data in the context of health and diseases [3,11]. Network medicine can be used to elucidate the molecular complexity of human diseases while offering computational methods to discern how such complexity controls disease manifestations, prognosis, and therapy [3]. Recent advances in systems biology have greatly expanded both its breadth and depth, with a host of new technologies and methods, both experimental and computational, for use with high-throughput data [2,8]. Furthermore, these approaches can be used to analyze the massive amounts of clinical data readily accessible through electronic medical records, with translational bioinformatics capable of integrating this biological and clinical information into a comprehensive picture of disease pathogenesis (Fig. 1) [7,12].
How to reconstruct a disease network and select key regulators
Complex diseases such as RA are the result of a combination of genetic and environmental perturbations rather than a single, easily identified cause. Systems biology approaches diseases such as this by reconstructing perturbed gene networks to identify key mediators of pathology. These types of investigation are usually conducted in a similar manner, with a typical systems-based study employing many of the same basic approaches [13]. First, investigators should define the "system," which can range in size from a single organelle to the entire organism, depending on the disease. The second step is to select the components involved in the system. Typical components include mRNA transcripts, noncoding RNAs, proteins, metabolites, or physiological or pathological parameters. Third, the investigators need to determine the degree of interaction between these components, often using in vitro experiments such as co-immunoprecipitation to define protein-protein interactions (PPIs). Alternatively, investigators can apply biological network maps using existing information related to these interactions, including PPI networks (Search Tool for the Retrieval of Interacting Genes/Proteins [STRING], Human Protein Reference Database [HPRD]), metabolic networks (Kyoto Encyclopedia of Genes and Genomes [KEGG], Biochemical Genetic and Genomic [BiGG]), RNA networks (TargetScan), and regulatory networks [3]. While public interaction databases cover a wide range of potential interactions, no list is exhaustive, with many interactions either too newly discovered or not yet established to be included in the database. In this case, investigators must either search published articles to find reported interactions, or use literature-mining tools such as Information Hyperlinked over Proteins [14]. The fourth step is to model the network mathematically to identify changes in interactions between network components in response to disease-related perturbations. Finally, investigators should validate the models experimentally.
Our recent study shows how the systems approach functions for investigation of a reconstructed RA-perturbed network [14]. First, RA-associated genes (RAGs) were selected by analyzing gene expression data sets generated from RA, osteoarthritis, and normal synovial tissues. These RAGs were reconstructed as an RA-perturbed network showing RA-associated cellular processes and the interactions between RAGs, using public protein interaction databases such as STRING and KEGG (Fig. 2A). This reconstructed network was used to identify the key cellular player in RA synovium, and demonstrated the effects of tumor necrosis factor α (TNF-α), interleukin 1β (IL-1β), and anti-TNF therapy on the genes and modules included in the networks. These target molecules, which can potentially modulate RA-perturbed networks, were selected based upon the number of interactions among the RAGs.
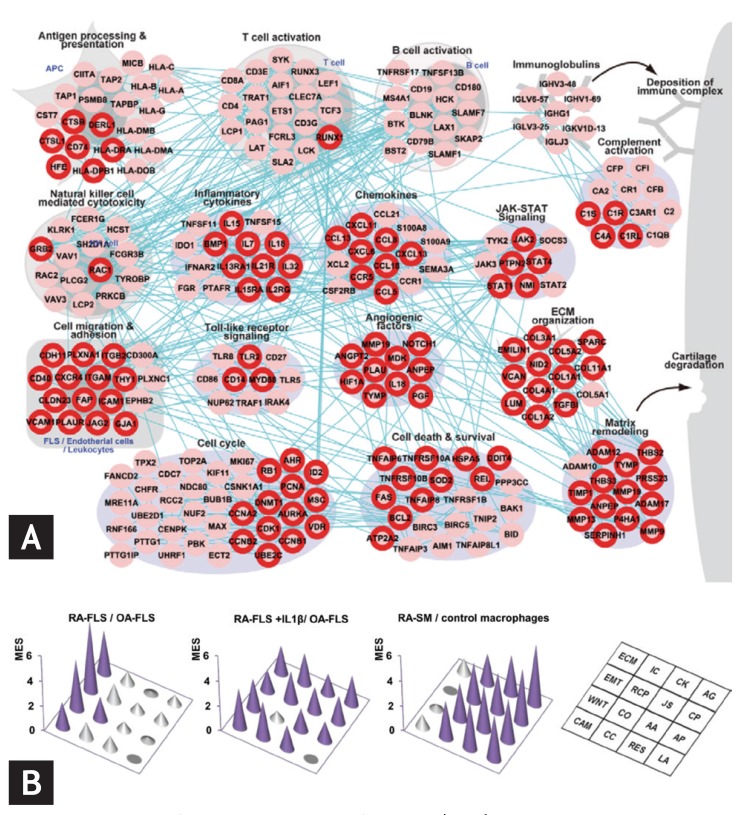
A rheumatoid arthritis (RA)-perturbed network in the RA synovium and enriched modules in the RA-fibroblast-like synoviocytes (FLS) and synovial macrophages (SM). (A) An RA-perturbed network describing RA-associated cellular processes, involving 242 upregulated RA-associated genes (RAGs), and their interactions. The network nodes are arranged into 16 modules based on their associated GOBP (Gene Ontology Biological Processes) and KEGG (Kyoto Encyclopedia of Genes and Genomes) pathways. The nodes with the red boundary represent differentially expressed genes (DEGs) in RA-FLS. (B) Three-dimensional conic graphs showing module enrichment scores (MES) representing the contribution of RA-FLS and SM to the individual modules. The three sets of MES were computed from the comparisons of RA-FLS and osteoarthritis (OA)-FLS (left), RA-FLS + interleukin 1β (IL1β) and unstimulated OA-FLS (middle), and RA-SM and control macrophages (right). The height of the circular cones indicates the magnitude of the MES in the corresponding module. Purple cones indicate MES p values < 0.01, whereas gray cones indicate MES p values > 0.01. Adapted from You et al. [14] and You et al. [15].
Systems approaches to diseases can also be used to identify functional characteristics of disease-associated cells and potential target molecules. In our recent study [15], a global transcriptome profiling of RA fibroblast-like synoviocytes (FLS) showed that differentially expressed genes (DEGs) in RA-FLS are enriched in key cellular processes related to cell invasion (Fig. 2B). A small group of genes was selected in modules related to the invasive potential of RA-FLS, among which we identifies a number of regulatory genes previously associated with RA pathogenesis, such as transcriptional regulators or signaling molecules.
Compelling results generated using the systems approach
Network analysis has to date presented compelling results which would not have been possible using traditional methods. The majority of disease-associated genes long considered central to disease pathology are, in fact, nonessential, and showed no tendency to encode hub proteins; moreover, their expression pattern indicated that they are localized on the periphery of the functional network [16]. This finding is significant, as it shows that many current drugs do not target the essential disease-associated proteins [17]; that is, most drugs are palliative and do not directly perturb the proteins corresponding to the underlying cause of disease. This observation may explain much of the unsatisfactory therapeutic efficacy in RA and other drugs, along with the variety of adverse effects associated with these treatments.
Disease modules from different disorders can overlap, with perturbations caused by one disease module directly affecting other disease modules. As a result, complex diseases often share similar phenotypic traits and comorbidities [18]. For example, RA, systemic lupus erythematosus (SLE), and diabetes are completely different diseases, but have an equally increased risk for accelerated vascular atherosclerosis. A more comprehensive study which modeled a network of disorders and disease-associated genes revealed significant clustering of many human diseases within the disease network, highlighting the common mechanisms underlying a collection of various human diseases that involve abnormalities in a specific, critical subset of genes [16]. The shared characteristics and interconnectedness of disease modules may explain why some drugs can treat many different diseases [19]. Furthermore, drug repositioning, the application of existing drugs for new indications, can be achieved on the basis common network targets [20,21], with a successful repositioning able to avoid the common pitfalls of drug discovery, including cost, high failure rates, the time necessary to bring a drug to market.
Points to be considered in systems analysis
Clearly, systems biology is an excellent tool for decoding large-scale multilevel data, and for understanding the relationship between genotypes and phenotypes in the context of health and diseases. However, this approach has several critical limitations. First, considerable progress has been made in technologies related to global cell measurements and the computational analyses associated with these investigations; however, these efforts remain costly and time consuming. Second, human interactome maps based on the published biological literature remain incomplete, with modules identified by analysis of high-throughput data less reliable than those derived from small-scale experimentation. Known disease genes tend to be investigated more extensively, which might introduce a bias toward higher connectivity. Moreover, many real and functionally relevant interactions are missing in the current PPI data sets. Third, network modeling does not replace any of the classical techniques of biochemistry or genetics; instead, it provides a set of organizing principles that integrate these data. In addition, interactions are often measured in a binary manner, with no information regarding the nature of an interaction. Computationally identified network modules typically lack a mechanistic explanation of pathway activities but rather serve as groups of genes that work together to achieve a particular function. The current estimates suggest that the human interactome comprises ~130,000 to 650,000 protein interactions [22,23]; however, only a subset of these has been determined experimentally. Therefore, the simulated physical and functional interactions should undergo a rigorous experimental validation process to elucidate their biological relevance. Fourth, both the nodes and the interactions discussed above must be evaluated in the context of tissue specificity. Fifth, a considerable portion of the reported results is interpretations of transcriptomic data from PPI analysis. However, mRNA expression accounts for only 40% of the variability in protein levels [24]. While the genome comprises 20,000 to 25,000 genes [25], the proteome is estimated to comprise > 1 million proteins [26]. Changes at the transcriptional and mRNA levels increase the size of the transcriptome relative to the genome, and the myriad of different posttranslational modifications exponentially increases the complexity of the proteome relative to both the transcriptome and the genome [27]. Finally, transcripts or proteins in the clinical samples can be affected by diverse clinical variables other than the disease itself. Attention must be given to the study design and patient selection to minimize the confounding effect of clinical factors, though biological variables are more difficult to control for than technical variables.
Systems approach and network analysis in rheumatic diseases
Systems biology approaches have only recently been employed in the study of rheumatologic diseases, with initial efforts focused on refining the existing knowledge, discovering novel biomarkers, and identifying new therapeutic targets for RA and SLE [28,29]. However, the majority of available data exist at the gene expression profiling and comparative analysis stages, with true data integration limited to a handful of major diseases. The detailed organizing principles, tools, and methods for understanding systems biology or network medicine are beyond the scope of this review; a series of recent reviews cover all of the relevant issues and methodologies in great detail [2,3,8,11,30]. Here, we will review recent efforts to use systems approaches for characterizing rheumatic diseases, and highlight key studies that have successfully integrated network theory, to afford new insight into the molecular and cellular interconnectedness underlying disease phenotypes.
Rheumatoid arthritis
RA is a good model for understanding the typical characteristics of complex diseases. RA is a chronic, progressive, debilitating autoimmune disease involving a multitude of environmental and genetic factors that show nonlinear dynamic interactions; moreover, it is highly dependent on sex and age [31]. The disease is characterized by chronic inflammation of the synovium, which over time results in irreversible damage to the joints, leading to pain and functional impairment. The proinflammatory cytokine TNF-α is a critical mediator of inflammation in RA [32]; the use of biological agents neutralizing TNF-α activity in combination with methotrexate has revolutionized the treatment of RA, producing significant improvements in clinical, structural, and functional outcomes [33,34]. However, TNF inhibitors are not effective in all patients; ~30% of patients treated with a TNF inhibitor failed to attain the treatment goal [35,36,37], while many more have discontinued treatment due to adverse effects or loss of efficacy due to secondary failure or acquired therapeutic resistance [38]. Moreover, in clinical practice, < 50% of patients were able to sustain remission for > 1 year [39], while ~50% of patients who reached drug-free remission restarted disease-modifying antirheumatic drugs because of relapse [40], a disappointing reality also seen with other biologics [41]. These results suggest that while TNF-α and IL-6 are key players mediating rheumatoid inflammation, they function primarily as intermediaries, with the control of one or two such cytokines insufficient to manage the disease. Given the heterogeneity among RA patients in terms of clinical outcomes despite otherwise similar phenotypes, further efforts are needed to discover more fundamental regulatory pathways and/or molecules governing the common pathologic traits, and to distinguish the role of individual genetic variation to guide personalized therapeutic intervention.
The most promising candidate genes associated with RA susceptibility were identified from large-scale GWAS and transcriptomic analysis [42]. An analysis of RA susceptibility genes using PPI databases showed the extent of potential physical interaction between the identified protein products. This network was built using RA candidate genes, and comprises major and minor network nodes. Several confirmed RA susceptibility loci such as TRAF6, STAT1, and IL2RB can be recognized among the major nodes [43]. Interestingly, CTLA4, currently an effective therapeutic target, and PTPN22, confirmed as a strong susceptibility gene, have fewer interactions than expected, and were positioned at the periphery of the network, inferring functional characteristics of genetic variants implicated in RA susceptibility. This hypothetical network was constructed using cumulated high-throughput data and PPI databases such as HPRD; however, the physical and functional interactions between proteins and their regulatory role in the modular processes of disease tissues and organs were not validated experimentally.
Another research group acquired extensive GWAS data by searching the electronic databases, and reanalyzing the data [44]. The risk and predictive value of each genetic variant for RA was assessed, and a PPI network was constructed using the HPRD database. Next, a random walk with restart algorithm was used to prioritize genes in terms of their proximity to validated RA susceptibility genes within the PPI network; ZAP70 was the top-ranked gene in this algorithm. Notably, a mutation in ZAP70 was shown to cause aberrant thymic T-cell selection in mice, leading to the development of RA-like symptoms [45]. ZAP70 has also been shown to directly interact with protein tyrosine phosphatase, non-receptor type 22 (PTPN22) and Fc receptor-like protein 3 (FCRL3) among the proteins encoded by RAGs in the HPRD database. Subsequent analyses revealed three functional modules in the RA-associated network, as determined using the agglomerativE hierarchicAl clusterinG based on maximaL cliquE (EAGLE) algorithm and Database for Annotation, Visualization, and Integrated Discovery (DAVID): leukocyte activation and differentiation, pattern-recognition receptor signaling pathway, and chemokines and their receptors. Taken together, these results demonstrated the utility of a systems genetics approach in shaping the understanding of biological pathways highlighted by the various common genetic variants associated with the disease. While the overarching results were largely simply a corroboration of existing knowledge regarding the molecular pathogenesis of RA, these findings lay the foundation for the mapping strategies necessary to elucidate biological pathways.
Recently, a study of RA patients was conducted examining gene expression profiles of whole blood collected before and during treatment with a TNF-α inhibitor. From these data, researchers were able to build a molecular network regulated by TNF-α, and identify the molecular signatures determining the heterogeneity of treatment response relevant to clinical index [46]. Twenty-two transcripts were identified to have new roles in modulating DAS28 scores, of which six represented potential targets of anti-TNF therapy. In particular, CD86 was identified as a strong modulator of DAS28, tender joints, or swollen joints, and represents an important TNF-α-independent mechanism active in RA patients. This finding is consistent with the therapeutic impact of modulating CD86 with abatacept (CTLA4-Ig) as an alternative to TNF-α inhibitors [47].
Disease modules are groups of nodes whose perturbations (mutations, deletions, copy number variations, or expression changes) can be linked to a particular disease phenotype, and can be found across different functional molecules [3]. Together, these modules make up a larger disease network, which is transformed by perturbation in one or more proteins within a module. Therefore, it is important to consider not only the effects on the individual module but also the larger disease network as a whole, depending on the disease status and treatment response. Our group developed a multifaceted systems approach for identifying core RAGs, reconstructing RA-perturbed networks, and selecting potential targets for the diagnosis and treatment of RA using expression data from 14 distinct gene sets, obtained from the synovial tissues, peripheral blood cells, and FLS of RA patients [14]. First, the RA-perturbed networks revealed that RA-FLS acts as a major factor in a wide range of RA-perturbed processes. Second, anti-TNF-α therapy causes a wide spectrum of RA-perturbed processes to tend toward normality; however, B cell-related modules, such as the B cell activation and the immunoglobulin modules, were only modestly affected by anti-TNF-α therapy, suggesting that B cell-targeted therapy may be effective for cases refractory to TNF-α inhibitors. Indeed, rituximab, an anti-CD20 monoclonal antibody, has been approved for the treatment of RA patients resistant to TNF-α inhibitors [48]. Finally, we identified a set of 19 key transcription factors (TFs) that play crucial roles in the regulation of 55% of the genes governed by RA-perturbed networks. Of note, we identified a set of candidate genes that had not previously been reported as diagnostic markers or therapeutic targets of RA, despite their strong association with RA-associated cellular processes (Fig. 3A). Of these candidate genes, we selected nuclear factor of activated T-cells 5 (NFAT5), known as an osmoprotective TF activated by hypertonicity, for further validation using human RA-FLS and heterozygous NFAT5-haplodeficient mice [49].
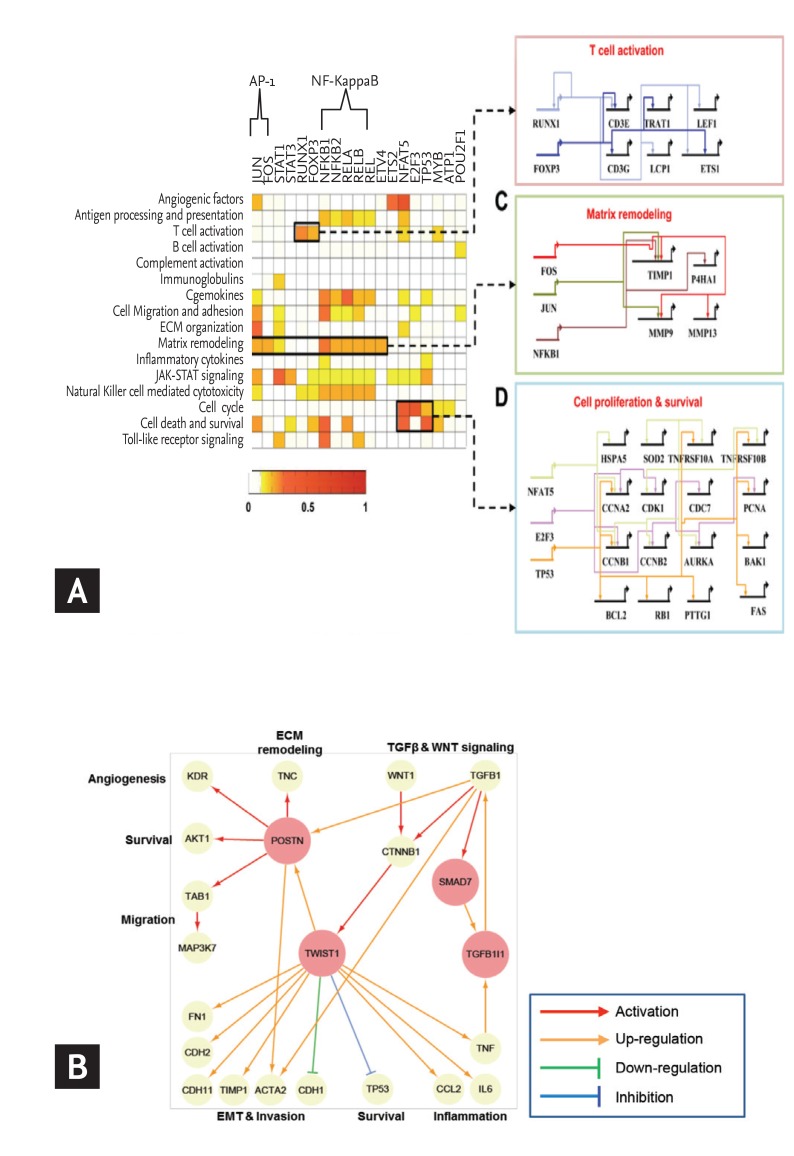
Network construction and identification of key regulators in rheumatoid arthritis (RA). (A) Gene regulatory networks activated in RA. Target enrichment scores representing the significance of overlaps between the targets of each transcription factor (TF) and the RA-associated genes belonging to the network modules. Gene regulatory networks describing the TF-target relationships for three processes: T-cell activation including Runt-related transcription factor 1 (RUNX1) and forkhead box P3 (FOXP3), matrix remodeling including activator protein 1 (AP-1) (JUN and FOS) and nuclear factor NF-kappa-B p105 subunit (NFKB1), and cell proliferation and survival including NFAT5, E2F3, and tumor protein p53 (TP53). (B) Selection of potential regulators for fibroblast-like synoviocytes (FLS) invasion. A network model describing the regulatory interrelationships of periostin (POSTN), twist family bHLH transcription factor 1 (TWIST1), mothers against decapentaplegic homolog 7 (SMAD7), Transforming growth factor beta-1-induced transcript 1 protein (TGFB1I1) and their associated processes. The arrows denote regulator-target gene relationships. NF-κB, nuclear factor-κB; IL-6, interleukin 6. Adapted from You et al. [14] and You et al. [15].
A previous analysis of process interactions gleaned from the publicly available microarray data identified NFAT5 as a major TF linking different modules in the center of a global interaction network [50]. In our analysis, we reconstructed a molecular network that featured FLS dominant cellular functions by comparing the transcriptomes of FLS and synovial macrophages (SM) under unstimulated and IL-1β-stimulated conditions. RA-FLS acquired a proinflammatory signature dominant in RA-SM without losing their inherent invasive properties [15]. From this network model, periostin (POSTN) and twist family bHLH transcription factor 1 (TWIST1) were identified as key regulators responsible for FLS invasiveness, with their functional significance was confirmed both in vitro and in vivo (Fig. 3B). Together, these efforts demonstrate the value of a systems-based approach for the study of complex diseases. This method provides a comprehensive and systematic basis for the mechanisms underlying RA pathogenesis, with the resulting RA-perturbed network models identifying a number of high-value targets for use as therapeutic targets and diagnostic markers.
Systemic lupus erythematosus
SLE is an autoimmune disease that affects predominantly females. In SLE, loss of tolerance to nucleic acids and their interacting proteins results in the production of pathogenic autoantibodies that cause inflammation and tissue damage [51]. Unlike RA, SLE has no specific target organ. The involvement of several organ systems during flare-up demonstrates the unusual heterogeneity of the disease, and presents a significant hurdle in terms of sample collection, deconvolution, and translation of high-dimensional data. Accordingly, most studies have focused on peripheral blood cells, investigating the association between the type I interferon (IFN) signature and disease activity indices.
The IFN signature has been associated with wide range of pathologies, including severe clinical manifestations in lupus nephritis (LN), disease activity indices, including the SLE disease activity index (SLEDAI) score, hypocomplementemia, and anti-dsDNA titer [52,53,54,55,56,57,58]; however, no correlation was observed in relation to disease severity or disease flare risk [56,58]. This limitation may be attributable to several factors such as clinical variability, including age, sex, comorbidity, and medication, the high dimensionality of the data, and the masking of transcript changes due to the relative abundance of bystander cells or a depletion of key effector cells [28]. In this regard, deconvolution of the data, subset analysis, and a module-based approach using unbiased, data-driven processes represent viable methods for addressing this problem [28,59,60]. In this regard, Chiche et al. [60] presented striking results based upon a modular transcriptional repertoire analysis. They examined microarray data obtained from 62 patients with SLE, revealing three specific IFN-related modules. These signatures were dynamic in nature, exhibiting a gradient of activation across samples, with distinct activation thresholds and patterns of upregulation for each module. Importantly, upregulation of individual IFN modules correlated with different patterns of clinical symptoms and biological marker expression. These data suggest that a more granular and focused approach to the various IFN signatures may represent a superior method for evaluating disease activity and/or prognosis, and may be used to tailor therapies targeting IFNs and related pathways.
Recently, a series of network-based analyses have been performed with the goal of identifying novel biomarkers or target molecules using data deposited in public repositories. Siddani et al. [61] studied immune networks in SLE, calculating centrality measurements based upon known protein interactions and signaling networks, identifying important disease-related candidate genes such as SOCS3, PDGRFA, NFKBIA, and NCF2, consistent with previous reports [62,63,64,65]. However, much follow up is necessary to validate these findings. In another study using the atBioNet database system, two major modules were generated from seed genes using a fast-network-clustering algorithm: inflammatory process and immune activity [66]. The major signaling pathway involved in the regulation of the genes in the inflammatory module was the mitogen-activated protein kinases pathway, whereas that involved in the immune activity module was the JAK-STAT signaling pathway. A total of 14 genes, five in the inflammatory module and nine in the immune activity module, have previously been identified as possible biomarkers for SLE. For instance, Gadd45a gene was reported to be associated with a lupus-like immune autoimmune response [67].
Murine models are a valuable tool for understanding human disease, as well as for identifying and testing drug candidates for subsequent human trials. Murine models mimic human inflammatory diseases; however, many of the responses seen in these models are only weakly predictive of the human condition [68]. LN is one of the most frequent and severe manifestations seen in at least 50% of patients with SLE [69]. Recent attempts have sought to identify the shared pathogenic mechanisms in the development of LN between mice and humans, and to determine which mouse model most accurately reflects specific molecular pathways occurring in humans [70]. To address this issue, gene-expression profiles from microdissected human LN kidney biopsies and whole kidneys from three SLE-prone murine models were compared. Both unique and common transcriptional networks were observed among the three murine models (NZB/W F1, NZW/BXSB, and NZM2410 mice) and human LN. The most commonly shared network nodes reflect the key pathologic processes of immune cell infiltration/activation, endothelial cell activation/injury, and tissue remodeling/fibrosis, with macrophage/dendritic cell activation as a dominant transcriptional pathway shared between the two species. These results highlight the pitfalls of extrapolating from a single mouse model to human disease, and vice versa; characteristics unique to each of the three murine models might be exploited to classify patients for further evaluations based on their renal molecular profiles [70].
Ankylosing spondylitis
Ankylosing spondylitis (AS) is a chronic, progressive disease characterized by inflammation of entheses, leading to new bone formation, syndesmophytes, and ankylosis of joints, primarily in the axial skeleton [71]. Zhao et al. [72] integrated an array of genomic data related to AS available from public repositories (Online Mendelian Inheritance in Man [OMIM] database, microarray experiments from the GEO database, and proteomic experiment results from the literature) to prioritize candidate AS disease genes in the context of human protein interactomes. Immune-mediated inflammation and imbalanced bone modeling were the two key pathologic processes in the network, with TNF-α and IL acting as the pivotal cytokines mediating this interaction. While this result corroborated the established hypothesis [73], it failed to produce novel insights.
Novel AS candidate genes were identified from whole blood transcriptional profiling using a whole-genome microarray approach. Pimentel-Santos et al. [74] validated 14 DEGs in AS patients, among which SPOCK2 (osteonectin) and EP300 were identified as interesting candidate genes that might be able to provide insight into AS progression. SPOCK2 has been shown to play a role in the regulation of bone remodeling [75,76] and has previously been implicated in AS pathogenesis [77]. A simulated network performed using this same data was relatively scattered and connected only loosely. Statistical analyses identified 11 genes with high topological scores along with two modules with functional significance. These two modules were enriched with majority of DEGs involved in cell receptor signaling, natural killer cell-mediated cytotoxicity, and primary immunodeficiency. However, the proposed genes and modules diverged substantially from the original study result [74], suggesting missing pieces in the puzzle of AS. Although several candidate genes, including RGS1, TLR4, sLIGHT, and BIP have been implicated in the pathogenesis of AS through microarray-based studies of peripheral blood mononuclear cells [78,79,80,81,82,83], gut cells [84], and synovial fluid mononuclear cells [85], their positions and interactions with other molecules were not considered within the network model. Systems analysis after full integration of the available data and/or deconvolution will be necessary to better elucidate these points.
CONCLUSIONS
Systems biology focuses on the coordinated, dynamic behavior of a group of molecules within entire biological systems, reinterpreting human diseases as the result of perturbations to the functional module or network. Systems biology provides a new perspective from which to explore the underlying molecular systems, which until now had been unattainable using existing methods. Furthermore, network-based approaches and modular modeling have the advantages of simulating the behavior of a biochemical molecule or system under different perturbations, or hypothetical conditions that may be of interest but not readily feasible in experimental settings. Such an approach can be used to streamline the traditional hypothesis generation mechanisms common to biochemical research, and reduce experimental trial and error by narrowing the predictive range to within functional modules. This approach may be useful for predicting the adverse effects of drugs, repurposing old drugs, and designing new drugs with maximal efficacy and minimal adverse effects [19,20,86].
The application of systems biology in clinical medicine is in its infancy. Systems approaches and network analysis are not scientific end points on their own, but instead valuable tools for the study of complex diseases. However, it is important to recognize that while a large number of multilevel biomolecular profiles have been stored and are continuously being updated, most provide only a snapshot of the biological network in selected cells, tissues, and organs, produced under limited experimental conditions and for a group of patients with similarities at a certain stage. The best way to assemble and integrate high-dimensional multiplatform data with high-level heterogeneity and temporal and spatial dynamics remains an open question [87]. In the future, we hope to see systems-based approaches and network analysis utilized in the study of a broad range of rheumatic diseases, allowing for a better understanding of the molecular and clinical features underlying the disease, and providing critical targets for screening and therapy.
Acknowledgments
This work was supported by grants from the Korea Healthcare Technology R&D Project, Ministry for Health, Welfare and Family Affairs (No. HI14C3417) and the National Research Foundation of Korea (NRF) funded by the Ministry of Education, Science and Technology (2014R1A2A1A11049812).
Notes
No potential conflict of interest relevant to this article was reported.