Fisher discriminant analysis of multimodal ultrasound in diagnosis of cervical metastatic lymph nodes in papillary thyroid cancer
Article information
Abstract
Background/Aims
The purpose of this study was to develop a diagnostic model utilizing multimodal ultrasound parameters to aid in the detection of cervical lymph node metastasis in papillary thyroid cancer (PTC) patients.
Methods
The study included 84 suspicious lymph nodes from 69 PTC patients, all of whom underwent fine needle aspiration with pathological results. Data from conventional grayscale ultrasound, shear wave elastography (SWE), and superb microvascular imaging were analyzed. Key ultrasound features were compared between benign and metastatic groups to create a diagnostic model using Fisher’s stepwise discriminant analysis. The model’s effectiveness was assessed with self-testing, cross-validation, and receiver operating characteristic curve analysis.
Results
Four features, namely lymphatic hilum (X1), cortical hyperechogenicity (X2), vascular pattern (X4), and SWEmean (X7), were integral to the discriminant analysis, resulting in the equation: Y1 = −3.461 + 2.423X1 + 0.321X2 + 1.620X4 + 0.109X7, Y2 = −8.053 + 0.414X1 + 2.600X2 + 2.504X4 + 0.192X7. If Y1 < Y2, the LN would be diagnosed as metastatic lymph nodes. The model demonstrated an area under the curve of 0.833, with a sensitivity of 83.33% and specificity of 83.33%.
Conclusions
The multimodal ultrasound diagnostic model, established through Fisher’s stepwise discriminant analysis, proved effective in identifying metastatic lymph nodes in PTC patients.
INTRODUCTION
In the past few decades, the incidence of thyroid carcinoma (TC) has continued to increase [1]. Papillary thyroid carcinoma (PTC) is the most common type of TC. Although mortality rates from thyroid cancer have remained relatively stable [2], but there are still 20–50% of PTC patients develop cervical lymph node metastasis (CLNM) [3]. The existence of CLNM is crucial for selecting neck surgical method and is an important risk factor for recurrence and patients’ mortality [4,5]. Consequently, accurate identification of CLNM is of great significance before surgery for TC patients. Ultrasound is the preferred imaging examination for diagnosis of TC and lymph node metastasis [6]. However, two-dimensional ultrasound has its limitations. A meta-analysis [7] showed the sensitivity of conventional ultrasound for diagnosis of CLNM was not excellent, especially for central compartment, the diagnostic sensitivity was to be 28% (95% confidence interval [CI], 21–36%). With the development of multimodal ultrasound such as high-frequency ultrasound, shear wave elastography (SWE), superb microvascular imaging (SMI) in recent years, the diagnostic sensitivity of new ultrasound technologies has greatly improved, and new ultrasound technologies have become the preferred imaging method for evaluating CLNM.
Among the various ultrasound-based techniques, SWE and SMI have emerged as powerful tools for detecting a range of thyroid diseases [8–10]. SWE is a medical imaging technique that assesses the elasticity or hardness of tissue by generating shear waves within the tissue through the emission of sound waves, and then tracking the speed of these waves using ultrasound. Because the speed of shear waves is correlated with the tissue’s elasticity, analyzing these waves provides valuable information about tissue hardness [11]. SMI, on the other hand, facilitates the visualization of slow-moving blood flow and offers a more detailed representation of vascular circulation. This technological advancement enables the acquisition of high-quality images of microvascular flow without the necessity of contrast media [12]. As a novel ultrasound imaging technology, SMI can depict low-speed blood flow and small blood vessels with high resolution and sensitivity, and it does so without relying on contrast agents. It is capable of evaluating the perfusion of micro blood flow within tissues and organs. SMI outperforms power Doppler ultrasound sonography in providing detailed information about nodal vessels by visualizing small nodal vessels that would otherwise be challenging to detect [13]. Both SWE and SMI have been utilized to differentiate lymph nodes with varying pathologies, demonstrating impressive performance in doing so [13–15].
While the utility of any single diagnostic feature is limited, combining multiple sonographic characteristics can enhance the thoroughness and effectiveness of diagnostic assessments. Thus far, investigations into the comparative effectiveness of the different ultrasound modalities, individually or in conjunction as part of a multimodal imaging strategy, for diagnosing cervical lymph node conditions in thyroid cancer patients are limited. Discriminant analysis, a multivariate technique, is used to differentiate between groups of interest and aids in identifying which variables contribute to or predict the classification of observations into distinct groups. This approach can be adapted for either diagnostic or predictive analyses, depending on the research objectives [16]. The objective of this study was to develop a diagnostic model that utilizes multimodal ultrasound characteristic parameters (including grayscale ultrasound, SMI, SWE) through discriminant analysis, aiming to furnish dependable insights for the identification of CLNM in PTC patients.
METHODS
Study population
This prospective study was conducted in accordance with the declaration of Helsinki and received approval from the Ethics Committee at The First Affiliated Hospital of Shandong First Medical University & Shandong Provincial Qianfoshan Hospital (YXLL-KY-2022[043]).
Patients who were diagnosed with TC and had undergone thyroidectomy with newly discovered suspicious lymph nodes between January 2022 and July 2023 were considered for inclusion in this study. The inclusion criteria were as follows: (1) Postoperative patients with PTC who develop new abnormal cervical lymph nodes. (2) The lymph nodes had successfully undergone fine needle aspiration (FNA) and obtained clear cytological diagnosis results. The exclusion criterion was lymph nodes without clear cytological results because of inadequate cytological specimen or inconclusive result. All participants in this study provided informed consent prior to their participation.
Ultrasound examinations
Conventional grayscale ultrasound, SWE, and SMI images were obtained using an Aplio i900 or i800 system (Canon Medical Systems Corporation, Tochigi, Japan) equipped with a linear array transducer with a bandwidth of 5 to 18 MHz. Two radiologists (YH and FL) with experience of 3 and 8 years in ultrasound performed lymph node examinations. Two or more grayscale images were acquired for each lymph node. We evaluated the size, location, echogenicity of the cortex and visualization of the hilar fatty tissue.
After that, SMI and SWE were performed with minimum pressure from the transducer. The mean value of the vascular index (VI) and SWE for each lymph node was calculated from three measurements of each node obtained from longitudinal views. The parameters of SMI were shown as follows: velocity scale was 1.5 cm/s. For qualitative analysis, vascular pattern of lymph node was observed and reported. For quantitative analysis, VI was measured at the image with the strongest blood signal. VI is the ratio of number of pixels of vascular signal to the whole lymph node (tracing the outline of lymph node) and was automatically calculated and displayed on the screen (Fig. 1A). Margin of each lymph node was manually traced and the outline was on behalf of region of interest (ROI). We then switched to SWE mode and stiffness of lymph node was measured. Shear waves generated from the probe and displayed in a real-time color map. The average stiffness of lymph node (SWEmean) was assessed by placing ROI (tracing the outline of lymph node) and was expressed in kPa (Fig. 1B).

Examples of measurement using SMI and SWE. Margin of each lymph node were manually traced and the outline was on behalf of ROI. (A) VI was the ratio of number of pixels of vascular signal to the whole lymph node (tracing the outline of lymph node) and was automatically calculated and displayed at the bottom. (B) Average elasticity (kPa) of ROI was displayed automatically. SMI, superb microvascular imaging; SWE, shear wave elastography; ROI, region of interest; VI, vascular index.
Sonographic features evaluation
According to 2021 Korean Thyroid Imaging Reporting and Data System [17], we selected suspicious lymph nodes. Lymph nodes with any of the following features are defined as suspicious: cystic areas, hyperechoic foci (calcifications), cortical hyperechogenicity (focal/diffuse), or abnormal vascularization (peripheral/diffuse). We carefully evaluated the ultrasound features of the lymph nodes, including the presence or absence of a lymphatic hilum, the presence or absence of cortical hyperechogenicity, the presence or absence of calcification, the presence or absence of cystic components (Fig. 2) and the vascular pattern. There are four acknowledged vascular patterns, including hilar, peripheral, mixed and avascular or spot vascularity (Fig. 3). Mixed or peripheral vascularity was considered a malignant feature.

Sonographic features of lymph nodes. (A) Lymphatic hilum (arrow) of a benign lymph node. Normal lymph nodes are oval, with a peripheral cortex hypoechoic to the strap muscles, with a central echogenic hilum. (B) Focal cortical hyperechogenicity (arrow) to the cortex with absence of the hilum in a metastatic lymph node. (C) Echogenic foci (microcalcifications) (arrows) in a metastatic lymph node. (D) An anechoic space (arrow) representing small cyst change in a metastatic lymph node.

Four kinds of vascular pattern by SMI. (A) Hilar vascular. (B) Peripheral vascular. (C) Mixed vascular. (D) Spot vascular. SMI, superb microvascular imaging.
Due to the subjective nature of ultrasound feature evaluation, two ultrasound physicians with more than five years of work experience independently reviewed the images and evaluated the features. Consistency analysis was performed on the results. The final ultrasound features of the lymph nodes were determined based on the results of these two evaluations. In case of disagreement, a third senior physician was consulted for a final determination.
FNA
Under the guidance of color ultrasound, puncture site was selected. After routine disinfection, a sterile drape was placed and 2% lidocaine was used for local anesthesia. A 23G biopsy needle (BN-MAR-1/GA1; GMT Medical, Beijing, China) was inserted into the lymph node avoiding blood vessels. The aspiration was performed for three times and smear was made. After that, smears were sent for cytological examination. To prevent complications, all patients were monitored for 30 minutes after the procedure and underwent an ultrasound follow-up before discharge.
Cytological examination
The gold standard of cytological diagnosis for distinguishing benign from metastatic lymph nodes were that thyroid follicular epithelial cells with papillary nuclear features were found in the cytological smears.
Statistical analysis
The normality of the distribution of the continuous data was verified using the Shapiro–Wilk tests. Patients were divided into two groups: benign and malignant. Continuous data (such as age, size, VI, and SWEmean) were expressed as mean ± standard deviation or median with range (interquartile range). The independent samples t-test or Mann–Whitney U test was used to compare the two groups. Percentages of categorical data were calculated and Fisher’s exact test or the χ2 test were used to calculate the significance of the difference of categorical variables such as sex, laterality and location of lymph nodes, grayscale ultrasound features between the metastatic group and benign group. The reproducibility of the ultrasound feature evaluation was tested using Kappa consistency analysis. The diagnostic capacity of parameters was evaluated using receiver operating characteristic (ROC) curve analysis. The diagnostic accuracy of parameters was showed by calculating the area under the ROC curve (AUC). We calculated sensitivity, specificity, positive and negative predictive values and diagnostic accuracy. Using ultrasound features as the independent variable and the pathologic diagnosis as the dependent variable, linear regression analysis was performed to diagnose multi-collinearity of ultrasound indicators with statistical significance (p < 0.05) between the benign group and the metastatic group. Variables that could be included in the model were selected, and further Fisher stepwise discriminant analysis was used to eliminate variables that cannot be included. Some ultrasound features were then selected through Fisher’s stepwise discriminant analysis. The stepwise process involves iteratively adding and removing variables based on their Lambda values. In each step of the stepwise discriminant analysis, the variable with the smallest Lambda proceeds to the next step. A comprehensive diagnostic model for metastatic lymph nodes based on multimodal ultrasound was established. And self-testing method and leave one cross validation were performed for testing and ROC curve was constructed to evaluate the effectiveness of the diagnostic model.
All statistical analysis was performed using SPSS (version 25.0; IBM Corp., Armonk, NY, USA) and MedCalc version 15.8 statistical software (MedCalc Software bvba, Ostend, Belgium). A p value < 0.05 was considered to indicate statistical significance.
RESULTS
Demographic data and sonographic features
A total of 84 lymph nodes from 69 patients were eligible for inclusion in this study, including 51 females and 18 males with a mean age of 43.6 years (range, 20–74 yr). All patients included in our study were diagnosed with PTC. Table 1 displays the characteristics lymph nodes. There were no significant differences in the location, size of lymph nodes between the two groups. Among the 48 benign lymph nodes, 16 (33.33%) presented with a lymphatic hilum, while only 1 of the 36 malignant lymph nodes (2.78%) did. Cortical hyperechogenicity was obsevred in 8 of 48 benign lymph nodes (16.67%) and 24 of 36 (66.67%) metastatic lymph nodes. Calcification was detected in 3 of 48 benign lymph nodes (6.25%) and 8 of 36 (22.22%) metastatic lymph nodes. Cystic area was found in 4 of 36 (11.11%) metastatic lymph nodes. The hilar vascularity was present in 32 of the 48 benign lymph nodes (66.67%), while 28 of the 36 metastatic lymph nodes (77.78%) showed peripheral or mixed vascular signal. All these differences were statistically significant (p < 0.05). The VI and SWEmean values of metastatic lymph nodes were significantly higher than those of benign lymph nodes (p = 0.002, p < 0.001). The cutoff values for VI and SWEmean were 12.9 and 21.4 kPa, respectively. The kappa coefficients for sonographic features (the presence or absence of a lymphatic hilum, cortical hyperechogenicity, calcification, cystic area, and vascular pattern) between the two observers were approximately 0.688, 0.845, 0.950, 0.851, and 0.950, respectively. This analysis demonstrates that the ultrasound features were evaluated with good reproducibility and consistency.
Diagnostic performance of different sonographic features
Figure 4 illustrates the AUCs for various sonographic features. Among the gray-scale sonographic features, the vascular pattern exhibited the highest AUC. The sensitivity, specificity, positive predictive value, negative predictive value, and accuracy of vascular pattern in SMI were 77.78%, 85.42%, 80.00%, 83.67%, and 82.14% respectively. Table 2 lists the diagnostic performance of various sonographic features in distinguishing between benign and metastatic lymph nodes.
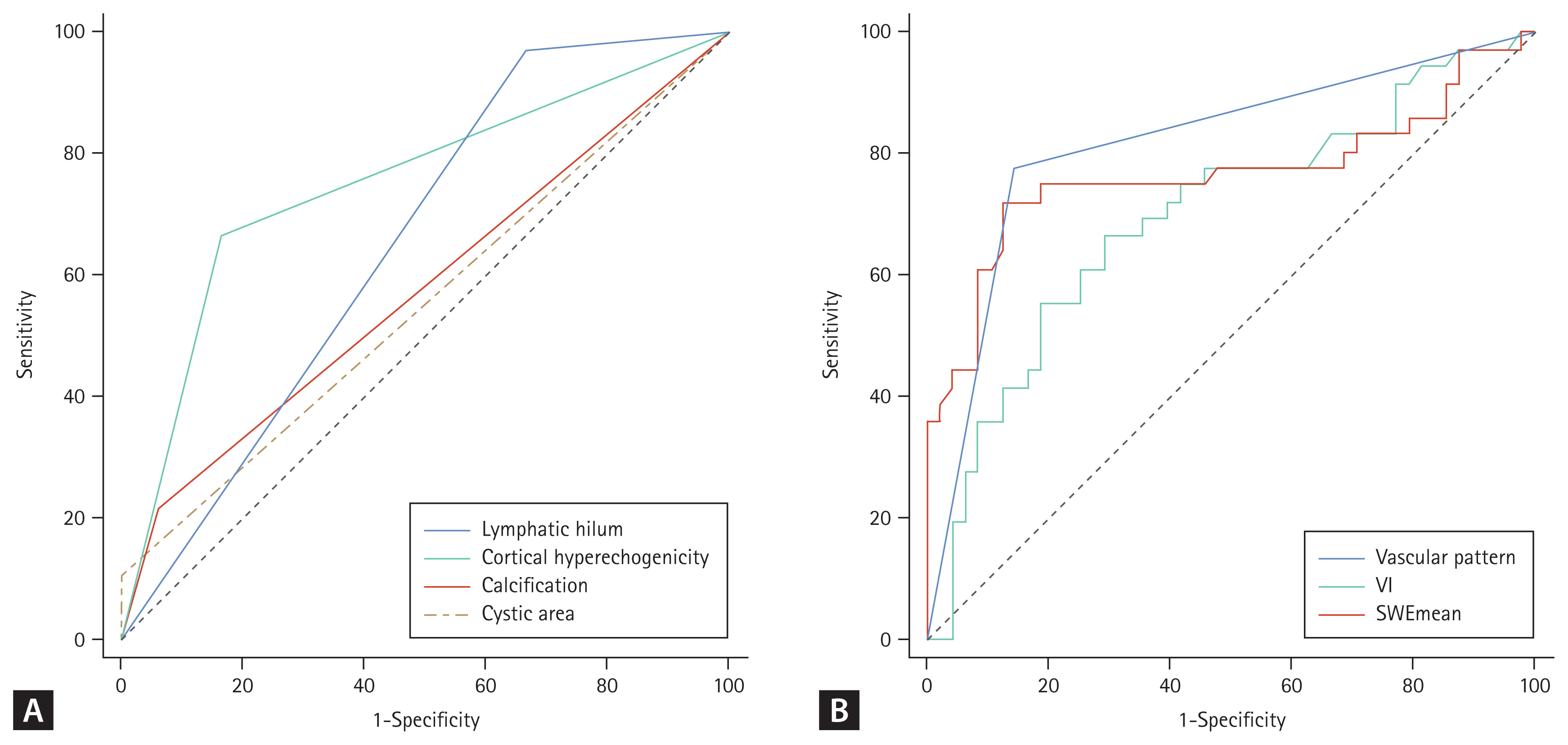
ROC analysis of grayscale ultrasound features (A) and SMI, SWE (B) in differentiating benign and metastatic lymph nodes. VI, vascular index; ROC, receiver operating characteristic; SMI, superb microvascular imaging; SWE, shear wave elastography.
Fisher stepwise discriminant analysis
The pathological type of lymph nodes was used as the dependent variable, and the features of multimodal ultrasound with statistically significant differences (p < 0.05) were assigned as independent variables (Table 3). To avoid strong linear relationships between variables, which may lead to inaccurate parameter estimation in the Fisher discriminant equation, collinearity diagnosis was performed before constructing the diagnostic model. The values of tolerance of all the variables were > 0.1, and the values of variance inflation factor were all < 10 (Table 4). Therefore, it was believed that there was no multi-collinearity between the above variables, and Fisher discriminant analysis can be used for the study. Furthermore, Fisher’s stepwise discriminant analysis was performed for analysis. The stepwise process involved iteratively adding and removing variables based on their Lambda values. In each step of stepwise discriminant analysis, the variable with the smallest Lambda would proceed to the next step (Supplementary Table 1). The standardized canonical discriminant function coefficients for the four selected variables, namely lymphatic hilum (X1), cortical hyperechogenicity (X2), vascular pattern (X4), and SWEmean (X7), have been calculated to determine the magnitude of each variable’s influence on the dependent variable. The order of the variables by their influence, from the most to the least, was as follows: SWEmean (X7), cortical hyperechogenicity (X2), vascular pattern (X4), and lymphatic hilum (X1). Discrimination equations based on their classification function coefficients were established. Benign group: Y1 = −3.461 + 2.423X1 + 0.321X2 + 1.620X4 + 0.109X7. Malignant group: Y2 = −8.053 + 0.414X1 + 2.600X2 + 2.504X4 + 0.192X7. If Y1 < Y2, the lymph node would be diagnosed as metastatic lymph node.
The diagnostic model was validated using both self-validation and leave one cross-validation methods, and the results showed high accuracy, with accuracy rates of 83.3% and 79.8% (Supplementary Table 2, 3). The diagnostic performance of the model was evaluated by constructing an ROC curve, and the results showed that the area under the curve was 0.833, with a 95% CI of 0.736–0.906. The sensitivity (83.33%) and specificity (83.33%) were both good (Fig. 5).
DISCUSSION
In this study, we utilized multimodal ultrasonic features of lymph nodes (including grayscale ultrasound, SWE and SMI) to construct a diagnostic model for identifying metastatic lymph node in PTC patients. Fisher’s stepwise discriminant analysis was employed and four sonographic features were ultimately included (vascular pattern, SWEmean, presentence or absence of cortical hyperechogenicity and lymphatic hilum). The multimodal ultrasound diagnostic model, established through Fisher’s stepwise discriminant analysis, proved effective in identifying metastatic lymph nodes in TC patients.
The American Thyroid Association recommends that all patients who undergo thyroidectomy for thyroid cancer confirmed by biopsy must undergo preoperative ultrasound examination to assess neck lymph nodes and as part of risk stratification for recurrence [18]. It has been reported that the sensitivity of punctate calcification and cystic changes in lymph nodes is usually low (less than 50%), and the specificity is relatively high (more than 90%). The sensitivity of lymphatic hilum disappearance was high (88% to 93%), and the specificity was not very bad (53% to 90%). The sensitivity of clustered high echogenicity was low, and the specificity was relatively high (sensitivity: 55% to 86%, specificity: 70% to 95.5%) [19,20]. This is consistent with our study results. In this study, the sensitivity of lymphatic hilum and cortical hyperechogenicity was 97.22% and 66.67%, respectively, and the specificity was 33.33% and 83.33%, respectively. The sensitivity of calcification and cystic changes in lymph nodes was 22.22% and 11.11%, respectively, and the specificity was 93.75% and 100% respectively.
Increased abnormal vascularity and altered vascular patterns in the lymph node are well-recognized imaging features of metastatic lymph nodes that have been widely acknowledged [17]. Hilar vascularity or absence of vascularity is typically observed in normal and reactive nodes while mixed or peripheral vascularity is invariably seen in metastatic or malignant lymph nodes [21,22]. Ahuja and Ying [23] found that the presence of peripheral vascularity, regardless of sole peripheral or mixed vascularity, was highly suspicious for malignancy. Similar to previous study, 85.42% of benign lymph nodes presented as one of hilar or spot vascularity, or absence of vascularity, while 77.78% of metastatic lymph nodes showed peripheral or mixed vascularity in this study. SMI is a novel type of ultrasound imaging technology that can visualize low-speed blood flow and small blood vessels with high resolution, high sensitivity, and without the need for contrast agents. It allows for the evaluation of microvascular perfusion within tissues and organs. In this study, we demonstrated the AUC for vascular pattern evaluated using SMI was the highest when evaluating lymph nodes using a single parameter.
Compared to vascular pattern, VI is a quantitative index that represents the ratio of the number of pixels of vascular signal to the entire lymph node area. In a previous study, VI values obtained via SMI were found to be useful in differentiating malignant lymphoma and acute lymphadenitis from normal lymph nodes [14]. In our study, VI values of metastatic lymph nodes were significantly higher than those of benign lymph nodes. However, VI was excluded in the final Fisher’s stepwise discriminant equations. In previous research, the hilar vascularity of malignant lymph nodes was observed to decrease due to lymphatic sinusoid obstruction and vascular displacement. Therefore, the overall vascular distribution of metastatic lymph nodes did not increase significantly as anticipated [14]. This may explain why VI was not as effective as vascular pattern in diagnosing metastatic lymph node. Yamaki et al. [24] proved that in the early stage of lymph nodes metastasis, tumor cells infiltrated and replaced microvascular areas, leading to perfusion defects. During the development of perfusion defects, the normal tissue was replaced by tumor, which was supported by only a limited number of micro vessels. This led to an increase in intranodal pressure [25], while pO2 levels remained stable and tumor-induced neovascularization was not observed [26,27]. This could be the possible underlying mechanism.
SWE has been proven to be a valuable imaging method for diagnosing malignant lymph nodes. The AUCs of SWE in the diagnosis of malignant lymph nodes ranged from 0.77 to 0.976. [28–30]. Adding SWE to conventional ultrasound improved the sensitivity of lymph node diagnosis (from 81.1% to 92.0%) but decreased its specificity (from 73.2% to 67.6%) [31]. In this study, we compared the elasticity of benign and metastatic lymph nodes and found that the elasticity of metastatic lymph nodes was stiffer than that of benign ones. This finding was similar to previous studies [30,32,33]. In metastatic lymph nodes, due to the invasion and proliferation of tumor, the stiffness of metastatic lymph node was higher [30]. However, previous study showed the hardness of lymph nodes might decrease when necrosis occurs in malignant lymph nodes [32].
Relying solely on a single ultrasound feature cannot accurately diagnose metastatic lymph nodes. With the extensive application of clinical databases, discriminant analysis has been utilized in many medical fields. This study applied Fisher’s stepwise discriminant analysis to the diagnosis of metastatic lymph nodes, establishing a discriminant model using four ultrasound features. Compared to using a single ultrasound feature, this model can significantly improve the accuracy of diagnosis. The discriminant function we constructed has a high area under the ROC curve of 0.833, surpassing all other indicators, indicating that the discriminant function holds greater value than a single indicator in diagnosing metastatic lymph nodes post-thyroid cancer surgery. This warrants further research.
There were some limitations in this study. First, this study was a single-center study, and the number of patients recruited in this study was small. Second, we only collected grayscale ultrasound, SMI and SWE features of lymph node. Other parameters such as quantitative analysis of contrast-enhanced ultrasound were not included. Third, only suspicious lymph nodes in patients were included in this study, while volunteers with normal lymph nodes did not included in our study. Although this model has shown high diagnostic efficiency, more external validation should be used to confirm its effectiveness.
In conclusions, the multimodal ultrasound diagnostic model in patients with PTC established using Fisher’s stepwise discriminant analysis was useful for differentiating benign from metastatic lymph node. Vascular pattern observed in SMI, the value of SWEmean, the presence cortical hyperechogenicity and absence of lymphatic hilum were the four most important features.
KEY MESSAGE
1. We conducted a Fisher’s discriminant analysis model to differentiate benign from metastatic lymph node in PTC patients.
2. Of the grayscale sonographic features, the AUC of vascular pattern was highest.
Acknowledgments
Special thanks go to the participants of this study, whose contributions were essential to the success of this research. Thanks to Dr. Fengjing Fan and Dr. Jing Fu for their assistance during the revision process of our manuscript.
Notes
CRedit authorship contributions
Yixuan Wang: resources, data curation, validation, software, writing - original draft; Yue Han: methodology, data curation, supervision; Fei Li: investigation, visualization, project administration; Yuyang Lin: investigation, supervision, project administration; Bei Wang: conceptualization, data curation, formal analysis, software, writing - review & editing
Conflicts of interest
The authors disclose no conflicts.
Funding
None