Derivation and validation of modified early warning score plus SpO2/FiO2 score for predicting acute deterioration of patients with hematological malignancies
Article information
Abstract
Background/Aims
Scoring systems play an important role in predicting intensive care unit (ICU) admission or estimating the risk of death in critically ill patients with hematological malignancies. We evaluated the modified early warning score (MEWS) for predicting ICU admissions and in-hospital mortality among at-risk patients with hematological malignancies and developed an optimized MEWS.
Methods
We retrospectively analyzed derivation cohort patients with hematological malignancies who were managed by a medical emergency team (MET) in the general ward and prospectively validated the data. We compared the traditional MEWS with the MEWS plus SpO2/FiO2 (MEWS_SF) score, which were calculated at the time of MET contact.
Results
In the derivation cohort, the areas under the receiver-operating characteristic (AUROC) curves were 0.81 for the MEWS (95% confidence interval [CI], 0.76 to 0.87) and 0.87 for the MEWS_SF score (95% CI, 0.87 to 0.92) for predicting ICU admission. The AUROC curves were 0.70 for the MEWS (95% CI, 0.63 to 0.77) and 0.76 for the MEWS_SF score (95% CI, 0.70 to 0.83) for predicting in-hospital mortality. In the validation cohort, the AUROC curves were 0.71 for the MEWS (95% CI, 0.66 to 0.77) and 0.83 for the MEWS_SF score (95% CI, 0.78 to 0.87) for predicting ICU admission. The AUROC curves were 0.64 for the MEWS (95% CI, 0.57 to 0.70) and 0.74 for the MEWS_SF score (95% CI, 0.69 to 0.80) for predicting in-hospital mortality.
Conclusions
Compared to the traditional MEWS, the MEWS_SF score may be a useful tool that can be used in the general ward to identify deteriorating patients with hematological malignancies.
INTRODUCTION
The survival of patients with hematological malignancies has recently improved because of advances in chemotherapy protocols and transplantation conditioning regimens [1,2]. Aggressive treatment and improved general care has also led to an increased demand for intensive care among hospitalized patients with hematological malignancies [1]. Favorable outcomes may be associated with an appropriate timing of transfer to the intensive care unit (ICU) [3], and previous studies have suggested that up to 50% of cardiopulmonary arrests in the general ward could be prevented by earlier treatment and transfer to the ICU [4]. Azoulay et al. [5] reported that earlier ICU admission was associated with improved survival in critically ill patients with hematologic malignancies. However, resource limitations in ICUs can lead to patients staying longer in the general ward and receiving inadequate treatment [6]. Thus, delayed ICU admission can lead to physiological deterioration, prolonged hospitalization, high costs, and poor outcomes [3,6,7].
Previous studies have revealed that the involvement of a medical emergency team (MET) can reduce the rates of unplanned ICU admissions, cardiac arrests, and in-hospital mortality [8,9]. The modified early warning score (MEWS) is used to identify patients in the general ward who will benefit from involvement of a MET, as well as to predict ICU admissions and in-hospital mortality [10- 12]. The MEWS tool is based on physiological variables that predict patient deterioration in high-risk cases and several studies have revealed that the MEWS can facilitate early ICU admissions, which results in better patient outcomes [10,11,13,14].
The National Institute for Health and Clinical Excellence [15] recommends routinely using a system for patient monitoring, such as the MEWS, in the hematology ward. This system should be able to provide early identification of at-risk patients and facilitate their referral to receive critical care before their deterioration. Although critical illness is characterized by physiological deterioration, there are few data to support the suggestion that the traditional MEWS can predict outcomes among patients with hematological malignancies [6,16,17]. Moreover, little is known regarding the effectiveness of the traditional MEWS for predicting outcomes among patients with hematological malignancies in the general ward. Therefore, the present study was conducted to evaluate the ability of the traditional MEWS to predict ICU admissions and mortality among patients with hematological malignancies in the general ward and to develop a modified MEWS based on these patients’ characteristics.
METHODS
Study cohort
This study was approved by the Institutional Review Board of Asan Medical Center, Korea (IRB no.: 2016- 0857). The need for informed consent was waived by the ethics committee as this study involved routinely collected medical data that were anonymously managed. This study enrolled two separate cohorts of deteriorating adult patients (aged ≥ 18 years) who received treatment from our MET in the general ward for hematological malignancies. All patients had been treated at the Asan Medical Center, which is a university-affiliated tertiary-care hospital in Seoul, South Korea. The center has approximately 2,700 beds, including 28 medical ICU beds, and treats approximately 100,000 adult patients each year.
The derivation cohort from which data were retrospectively collected included 220 deteriorating adult patients who received treatment from our MET in the general ward for hematological malignancies between January 2014 and November 2015. The validation cohort from which data were prospectively collected and retrospectively analyzed included 320 deteriorating adult patients in the hematological general ward between March 2016 and September 2017 (Fig. 1). The exclusion criteria were MEWSs that could not be calculated because of missing data, the implementation of not-for-resuscitation (NFR) before MET contact or within 24 hours after MET contact, and the MET being contacted to perform cardiopulmonary resuscitation (CPR).
The medical emergency team
The MET nurse practitioners, medical ICU residents, fellows, and staff performed MET activities daily if pre-defined vital signs or the laboratory threshold were reached by EMR monitoring or if a general word nurse or resident called the MET by telephone or pager [18]. In our hospital, the MET decided whether to transfer deteriorating patients to the ICU after initial assessment and treatment in the ward.
Data collection
Data were collected using case forms that were completed by the MET nurse practitioners and the patient’s electronic medical records in both the derivation and validation cohorts. Variables were the demographic data, types of malignancies, comorbidities, disease status, bone marrow transplantation (BMT) or hematopoietic stem cell transplantation (HSCT) and type (autologous versus allogeneic), presence of graft-versus-host disease, presence of febrile neutropenia, performance status [19] at admission, reason for MET activation, physiological data, laboratory data, quick sequential organ failure assessment (qSOFA) score, sequential organ failure assessment (SOFA) score, NFR after MET activation, and MEWS. The traditional MEWS consisted of the systolic blood pressure, pulse rate, respiratory rate, body temperature, and mental status [11]. The components of the qSOFA [20], SOFA [21], and MEWS [11] are shown in Supplementary Table 1.
The SpO2/FiO2 (SF) ratio was derived noninvasively using pulse oximetry [19,22]. The SF ratio was scored based on a previous study [22]: SF scores of 0 points for > 315, 2 points for 236 to 315, and 3 points for ≤ 235. The primary outcome of this study was ICU admission. The secondary outcome was in-hospital mortality.
Statistical methods
Data are expressed as medians with interquartile ranges. Statistical analysis of the data was performed using chisquare analysis for categorical data and nonparametric Wilcoxon tests for continuous data, as appropriate.
Univariate and multivariate logistic regression analyses were used to assess the MEWS and SF score as predictors of outcomes (ICU admission and in-hospital mortality). The multivariate analyses were adjusted for age, gender, comorbidities, underlying malignancies, disease status at admission, BMT recipients, performance status, the SOFA score, the qSOFA score, the reason for MET activation and NFR after MET activation, the MEWS, and the SF score. The results of these analyses were reported as odds ratios (ORs) with 95% confidence intervals (CIs).
Areas under the receiver-operating characteristic (AUROC) curves were calculated to evaluate the abilities of the MEWS, SF score, and MEWS plus SF (MEWS_SF) score to predict ICU admissions and in-hospital mortality. In these analyses, AUROC values of > 0.8 indicated good discrimination, values of 0.6 to 0.8 indicated moderate discrimination, and values of < 0.6 indicated poor discrimination [23]. We also calculated the sensitivities and specificities for predicting ICU admissions and in-hospital mortality.
Next, the prediction power of the MEWS, SF score, and MEWS_SF score were reassessed in the validation cohort. Differences with a p value of < 0.05 were considered statistically significant. All analyses were performed using IBM SPSS software version 20.0 (IBM Corp., Armonk, NY, USA).
RESULTS
Derivation cohort
A total of 2,832 deteriorating ward patients had MET contact during the derivation period, and 243 patients with hematologic malignancies were included. Eighteen patients who received NFR after MET contact, and 5 patients who were MET-activated by CPR were excluded. Finally, 220 patients were enrolled in the derivation cohort.
The characteristics at admission of these 220 patients are shown in Table 1. The median patient age was 54 years (interquartile range [IQR], 42 to 63), and 61.4% were male. The most common hematological malignancies were acute myeloid leukemia (41.3%) and lymphoma (23.6%). The disease status at admission was relapsed or refractory in 64.1% of the patients.
Table 2 describes the laboratory and clinical outcomes at MET contact. The main reasons for MET contact were respiratory distress (55.5%), sepsis or septic shock (26.8%), and altered mental status (4.5%). The median MEWS and SF score were 6 (IQR, 4 to 7) and 2 (IQR, 0 to 3). The ICU admission rate and in-hospital mortality rate were 46.8% and 51.8%%, respectively.
The univariate analyses revealed that ICU admission was significantly associated with BMT/HSCT recipients (OR, 1.89; 95% CI, 1.10 to 3.25), the MEWS (OR, 1.89; 95% CI, 1.57 to 2.28), the SF score (OR, 1.59; 95% CI, 1.30 to 1.95), and the SOFA score (OR, 1.12; 95% CI, 1.02 to 1.24) (Supplementary Table 2). The multivariate analyses revealed that ICU admission was independently associated with the MEWS (OR, 2.20; 95% CI, 1.67 to 2.78) and SF score (OR, 2.11; 95% CI, 1.47 to 3.02) (Table 3).
The AUROC curves for predicting ICU admissions using the MEWS, SF score, and MEWS_SF score are shown in Fig. 2. The AUROC values were 0.69 for the SF score, 0.81 for the MEWS, and 0.87 for the MEWS_SF score; both the MEWS and MEWS_SF score were considered to have good discriminatory value. The cut-off value for predicting ICU admission using the MEWS was 6 (sensitivity: 77.7%, specificity: 74.4%, positive predictive value: 72.7%, negative predictive value: 79.1%), and the cutoff value using the MEWS-SF score was 7 (sensitivity: 83.5%, specificity: 80.3%, positive predictive value: 79.0%, negative predictive value: 84.7%) (Table 4).
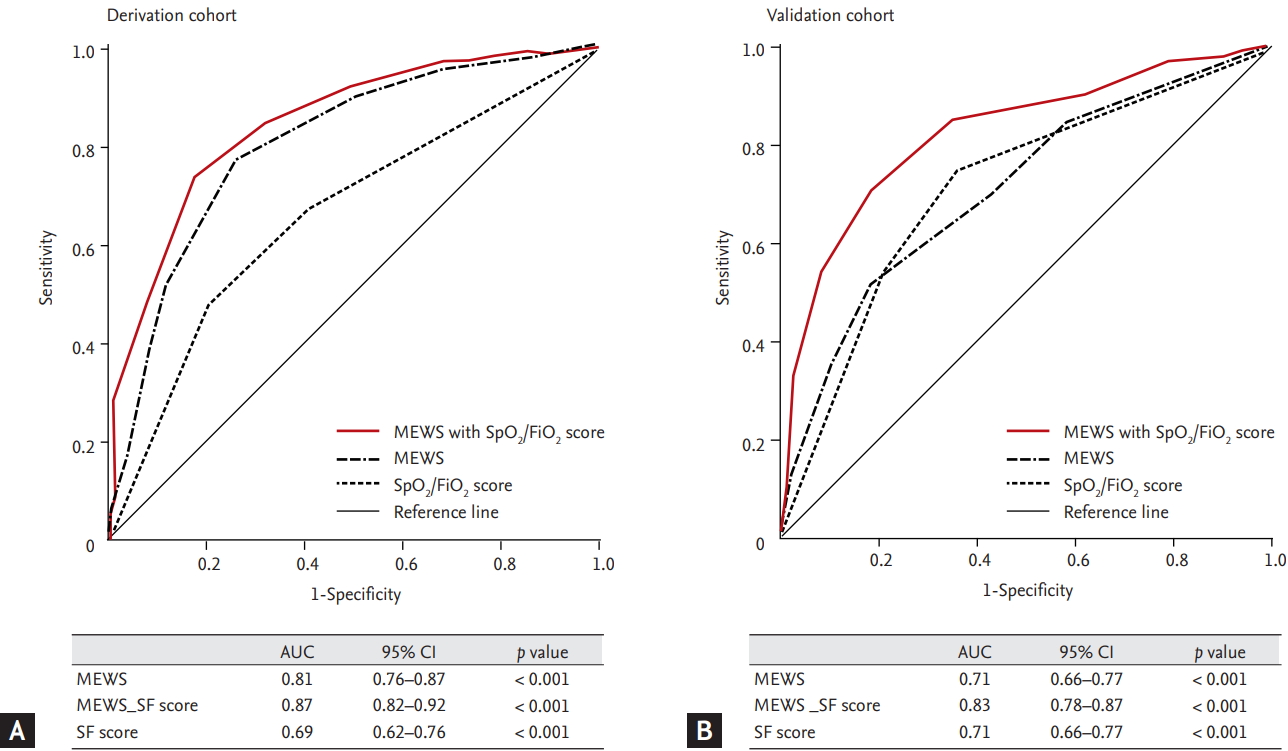
The receiver operator characteristic curves of modified early warning score (MEWS) and MEWS plus SpO2/FiO2 to predict intensive care unit admission after medical emergency team activation (SpO2/FiO2 scores of 0 points for > 315, 2 points for ≤ 315 SpO2/FiO2 ratio < 235, 3 points for ≤ 235). (A) Derivation cohort. (B) Validation cohort. AUC, area under the curve; CI, confidence interval; SF score, SpO2/FiO2 score.
The univariate analyses revealed that in-hospital mortality was significantly associated with respiratory distress (OR, 2.08; 95% CI, 1.21 to 3.60), the MEWS (OR, 1.34; 95% CI: 1.20 to 1.57), the SF score (OR, 1.80; 95% CI, 1.45 to 2.22), the SOFA score (OR, 1.13; 95% CI, 1.02 to 1.24), and NFR after MET activation (OR, 2.00; 95% CI, 1.04 to 3.81) (Supplementary Table 3). The multivariate analyses revealed that ICU admission was independently associated with the MEWS (OR, 2.01; 95% CI, 1.48 to 2.72) and SF score (OR, 2.01; 95% CI, 1.48 to 2.72) (Table 5). The AUROC curves for predicting in-hospital mortality using the MEWS, SF score, and MEWS_SF score are shown in Fig. 3. The AUROC values were 0.69 for the SF score, 0.70 for the MEWS, and 0.76 for the MEWS_SF score. The cut-off value for predicting in-hospital mortality using the MEWS was 6 (sensitivity: 70.2%, specificity: 71.7%, positive predictive value: 72.7%, negative predictive value: 69.1%), and the cut-off value using the MEWS-SF score was 7 (sensitivity: 71.9%, specificity: 60.4%, positive predictive value: 66.1%, negative predictive value: 66.7%) (Table 6).
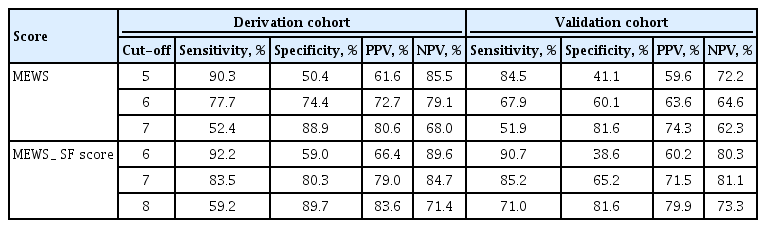
Sensitivity and specificity according to cutoff of risk score for intensive care unit admission in derivation and validation cohort
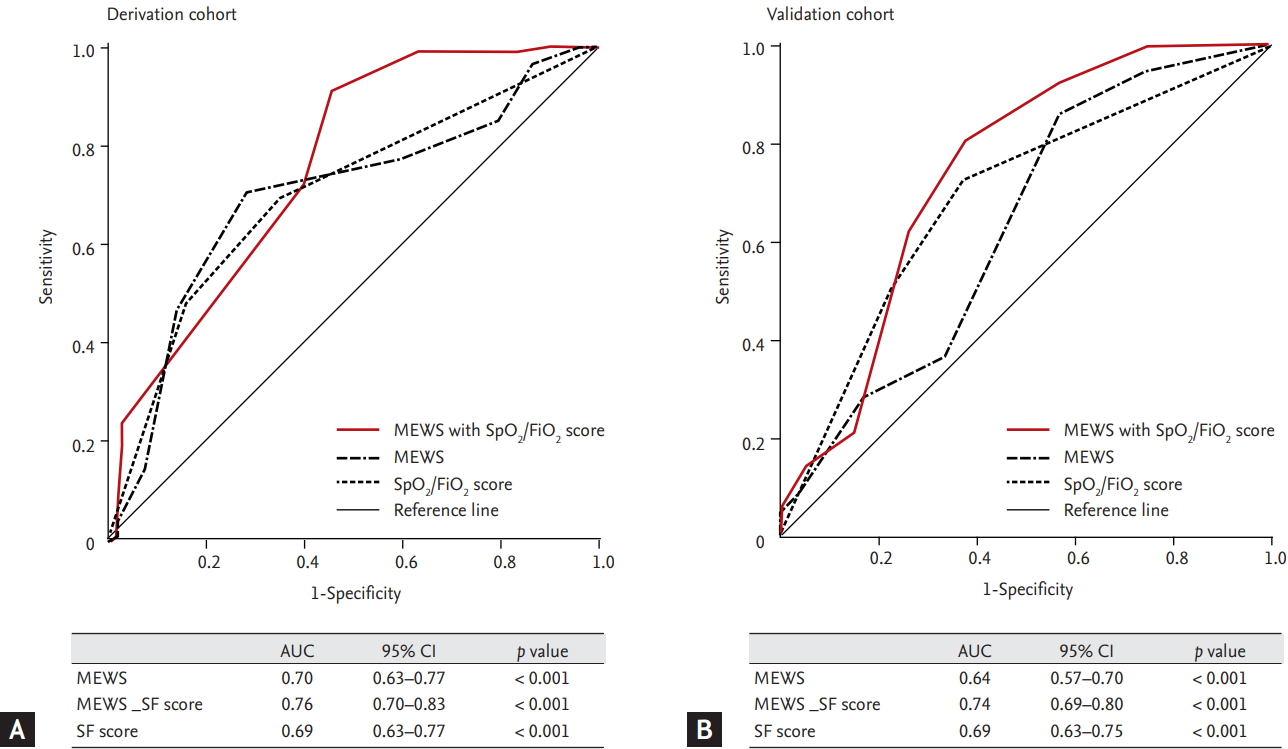
The receiver operator characteristic curves of modified early warning score (MEWS) and MEWS plus SpO2/FiO2 to predict in-hospital mortality after medical emergency team activation (SpO2/FiO2 scores of 0 points for > 315, 2 points for ≤ 315 SpO2/FiO2 ratio < 235, 3 points for ≤ 235). (A) Derivation cohort. (B) Validation cohort. AUC, area under the curve; CI, confidence interval; SF score, SpO2/FiO2 score.
Validation cohort
A total of 3,240 deteriorating ward patients had MET contact during the validation period, and 350 deteriorating ward patients with hematologic malignancies were included. Twenty-four patients who received NFR before MET contact and six patients who were MET-activated by CPR were excluded. We evaluated this separate cohort of 320 patients to test the validity of the MEWS_ SF score. Only the Eastern Cooperative Oncology Group performance status and rate of NFR after MET activation showed significant differences between the derivation and validation cohorts.
In the validation cohort, the AUROC curves for predicting ICU admissions using the MEWS, SF score, and MEWS_SF score are shown in Fig. 2. The AUROC values were 0.71 for the SF score, 0.71 for the MEWS, and 0.83 for the MEWS_SF score. The MEWS_SF score was considered to have good discriminatory value compared to the MEWS. The cut-off value for predicting ICU admission using the MEWS was 6 (sensitivity: 67.9%, specificity: 60.1%, positive predictive value: 63.6%, negative predictive value: 64.6%), and the cut-off value using the MEWS_SF score was 7 (sensitivity: 85.2%, specificity: 65.2%, positive predictive value: 71.5%, negative predictive value: 81.1%) (Table 4).
The AUROC curves for predicting in-hospital mortality using the MEWS, SF score, and MEWS_SF score are shown in Fig. 3. The AUROC values were 0.69 for the SF score, 0.64 for the MEWS, and 0.74 for the MEWS_SF score. The cut-off value for predicting in-hospital mortality using the MEWS was 6 (sensitivity: 62.1%, specificity: 55.0%, positive predictive value: 60.7%, negative predictive value: 56.5%), and the cut-off value using the MEWS_SF score was 7 (sensitivity: 80.5%, specificity: 62.3%, positive predictive value: 70.5%, negative predictive value: 74.0%) (Table 6).
DISCUSSION
The present study revealed that the MEWS_SF score provided an improved ability to predict ICU admission and in-hospital mortality, compared to the traditional MEWS, among deteriorating patients in a hematology ward. In this study, we evaluated the effectiveness of the MEWS among this specific high-risk population and modified the MEWS based on the characteristics of patients who are at risk of deterioration in the general hematology ward. Multivariate logistic regression analysis revealed that both the SF score and MEWS are independently associated with ICU admissions and in-hospital mortality.
Several previous studies have shown that > 30% of patients with hematological malignancies develop pulmonary complications and approximately 50% of these patients are admitted to an ICU [1,5,24,25]. Other studies have identified acute respiratory failure as a common reason for ICU admission among deteriorating patients with hematological malignancies [26,27]. Furthermore, previous studies have revealed that tachypnea, oxygenation parameters, and the PaO2/FiO2 ratio predict ICU admissions and mortality among patients with hematological malignancies [28,29]. However, arterial blood gas analysis in these patients with coagulopathy is difficult to perform routinely. The SF ratio provides a non-invasive measure of hypoxemia severity and has been proposed as a substitution for the PaO2/FiO2 ratio [22,30]. Sanz et al. [30] suggested that SpO2 may be sufficient for estimating PaO2/FiO2, and an early assessment of oxygen status using the SF ratio can be used to screen for patients that should be admitted to the ICU. The present study showed that the most common reason for ICU admission is respiratory distress requiring oxygen support; thus, we suggest that the SF score can be used to identify deteriorating patients in the general ward.
Mulligan [31] evaluated 71 patients with hematological malignancies in the general ward using two tools: the “trust tool” (an observation chart in which a single abnormal parameter is used to identify at-risk patients) and early warning score (EWS) that was developed by Morgan et al. [32]. The EWS was the first reported track and triggering system (T&TS), and many T&TSs have subsequently been developed (i.e., the MEWS) [16]. Mulligan’s revealed that the Trust tool and EWS (using a trigger score of 3) have high sensitivities (both 82%), but low specificities (7% and 17%, respectively). Cooksley et al. [33] reported that the current T&TSs (the MEWS used at the Christie Hospital and National Early Warning Score) have poor discriminatory values for identifying patients with malignancies who are at-risk of deteriorating and requiring ICU admission. Moreover, several studies have shown that improved sensitivity and specificity for the MEWS could be achieved by modifying the measure reflecting the patients’ characteristics and settings [6,15,16]. Because the findings of previous studies may have limited applicability to patients with hematological malignancies, we herein compared and analyzed the MEWS and SF score to improve the predictive power of ICU admission in this specific patient group.
We modified the traditional MEWS using the SF score as an oxygenation index, given that respiratory failure is the main cause of ICU admissions among patients in the hematology ward. In our study, the estimation of FiO2 in patients with spontaneous breathing was difficult. Indeed, FiO2 depends not only on the oxygen flow rate, but also on several factors such as the respiratory rate, tidal volume, device used to deliver oxygen, and oxygen leaks [34]. However, because our study population comprised deteriorating ward patients who were not using a ventilator, they could not undergo correct FiO2 measurements (they used a variety of O2 devices, such as a nasal prong, venturi-mask, simple mask, etc.) Our study used the SpO2/FiO2 score, which was validated as a noninvasive surrogate of the P/F ratio in mechanically ventilated patients in the ICU setting [22]. To minimize limitations, we performed a prospective validation study using a separate cohort. Our findings indicate that, compared to the traditional MEWS, the MEWS_SF score had a better ability to predict ICU admissions among patients with hematological malignancies.
The present study has several limitations. Our study was undertaken at a single center, which may have been associated with known risks of bias. Transfer to the ICU might have been affected by the attending clinician or occupancy rate of the ICU. Additionally, in-hospital mortality might have been affected by several factors, such as severity, disease status, and NFR after MET activation. However, we included and NFR after MET activation. However, we include the validation cohort to minimize these issues. Moreover, we performed multivariate analysis adjusted for baseline characteristics including severity, disease status, performance status, BMT/HSCT recipients and NFR after MET activation to improve causality.
In conclusion, the MEWS_SF score is superior to the traditional MEWS for identifying patients with hematological malignancies in the general ward who are at-risk for requiring intensive care and predicting in-hospital mortality. These findings suggest that oxygen monitoring is important in at-risk patients with hematological malignancies, and the MEWS_SF score may be a useful screening tool for determining ICU transfer and predicting in-hospital mortality in deteriorating patients with hematological malignancies.
KEY MESSAGE
1. The modified early warning score plus SpO2/ FiO2 (MEWS_SF) score is superior to the traditional MEWS to identify patients with hematological malignancies in the general ward who are at-risk for requiring intensive care.
2. The MEWS_SF score is superior to the traditional MEWS to predict in-hospital mortality in deteriorating ward patients with hematological malignancies.
3. The MEWS_SF score may be a useful screening tool to determine intensive care unit transfer, and predict in-hospital mortality in deteriorating patients with hematological malignancies.
Notes
No potential conflict of interest relevant to this article was reported.
Acknowledgements
This study was supported by a grant of the Korea Health Technology R&D Project through the Korea Health Industry Development Institute (KHIDI), funded by the Ministry of Health and Welfare, Republic of Korea (grant number: HI15C1106H).